Neuro-symbolic AI is often envisioned as the next generation, ‘third wave’ of AI that will offer unique advantages both in design-time (e.g., engineering effort and verifiability of results) and at run-time (e.g., performance and safety). While these claims are expected to be true for engineered systems where development processes are built on the model-based (symbolic) tradition, they have not been substantiated in convincing, scaled examples. Because the ISR domain is complex and challenging, our goal is to facilitate major advances in the design, implementation, and assurance of a set of relevant Neuro-Symbolic Components (NSCs) and systems that enable unaided ISR missions
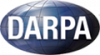
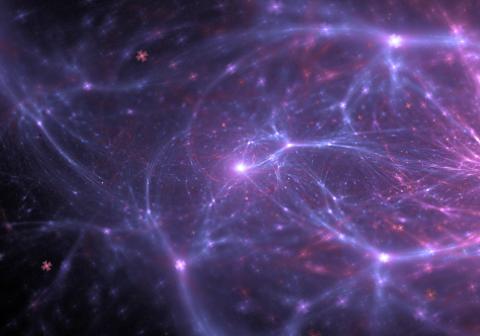
In “Algorithms and Architectures” the project focuses on
- defining a NSC Component Model that serves as the foundation for architecting complex applications, like the autonomy software stack for ISR systems,
- defining interfaces for integrating NSCs into system level functional and assurance architectures, where uncertainty in the behavior of NSCs needs to be managed,
- devising methods for the design-time and run-time composition of mission-specific functional and assurance architectures incorporating NSCs,
- developing novel NSCs for specific functions in an ISR application: mission planning and execution, and overall COP formation and dissemination,
- evaluating the design-time and run-time performance, safety and uncertainty characteristics of such NSC and architectures.
In “Specification and Assurance” the project focuses on
- developing methods for the specification and automated generation of challenging mission scenarios for training NSCs and for producing statistical evidence for assurance claims,
- defining the methods for characterizing NSCs for inclusion in an assurance framework,
developing a generic, probabilistic reasoning framework for the design-time composition of assurance arguments for systems with NSCs, - extending the framework for run-time use to support online dynamic risk management,
- applying the above specification and assurance tools in an autonomous Intelligence, Surveillance and Reconnaissance (ISR) context where the ultimate goal is the generation of a Common Operating Picture (COP), while assuring mission specific safety and performance requirements.
The effort, if successful, will expand the portfolio of Learning-Enabled Components (LECs) with novel capabilities that can be instantiated with moderate engineering efforts and solve typical ISR problems more efficiently and effectively than current approaches that rely exclusively on learning-enabled or on solely symbolic/algorithmic solutions. We anticipate significant reductions in the training time for the neural components, increase in robustness to noise and adversarial effects, and the confidence in the performance of the system. It is expected that the results: algorithms and tools will be widely applicable in a range of systems.