Anomaly detection, prognostication and automated mitigation are very critical for data center management. Most of these approaches can be divided into two categories - model-based and data-driven. While model-based techniques rely on physics guided models that can explain and predict the expected progression of parameters such as temperature and voltage in electronics, the data-driven approach is suitable for complex scenarios where a suitable physics based model is unavailable. The data-driven approaches can be further divided into supervised and unsupervised methods. While supervised methods rely on prior labels, unsupervised methods are more suited for cases where the prior failure data might be unavailable or where the failure trends change over time. This is precisely the problem that exists in modern data-centers where the network devices and models change over time and the prior labels are absent. The precise problem therefore is to develop unique anomaly scoring functions that can identify whether the various components in a complex device are failing or not. Note that unlike the prior art it is crucial to not only identify anomalies, but identify the sub-components at the silicon level which are failing in the devices.
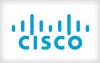
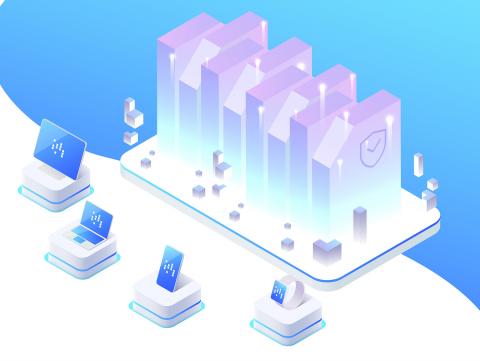