SI2-SSE: Deep Forge: a Machine Learning Gateway for Scientific Workflow Design
Recent advances in machine learning have already had a transformative impact on our lives. However, astonishing successes in diverse domains, such as image classification, speech recognition, self-driving cars and natural language processing, have mostly been driven by commercial forces, and these techniques have not yet been widely transitioned into various science domains. The field is ripe for innovation since many science fields have readily available large-scale datasets, as well as access to public or private compute infrastructure capable of executing computationally expensive artificial neural network (ANN) training workflows. The main roadblocks seem to be the steep learning curve of the ANN tools, the accidental complexities of setting up and executing machine learning workflows, and the fact that finding the right deep neural network architecture requires significant experience and lots of experimentation. DeepForge overcomes these obstacles by providing an intuitive visual interface, a large library of reusable components and architectures as well as automatic software generation enabling domain scientist to experiment with ANNs in their own field. There is unmet high demand of talent in machine learning, exactly because it has so much potential in a wide variety of application areas. Therefore, any tool that helps scientists apply machine learning in their own domains will have a broad impact. The promise of DeepForge is to flatten the learning curve, hide low level unimportant details and provide components that are reusable within and across disciplines. Therefore, DeepForge will have transformative impact on a number of fields.
DeepForge, a web- and cloud-based software infrastructure raises the abstraction of creating ANN workflows via an intuitive visual interface and by managing training artifacts. Hence, it enables domain scientists to leverage recent advances in machine learning. DeepForge will also integrate with existing cyberinfrastructure, including private and commercial compute clusters, cloud services (e.g. Amazon EC2), public supercomputing resources, and online repositories of scientific datasets. The DeepForge visual language for designing ANN architectures and workflows is powerful enough to capture the concepts related to common deep learning tasks, yet it provides a high level of abstraction that shields the users from the underlying complexity at the same time. DeepForge will provide a facility that allows for sharing design artifacts across a wide interdisciplinary user community. Curating a rich library of reusable components, integrating with a wide variety of existing cyberinfrastructure resources from data sources to compute platform and providing data provenance in a seamless manner are other advantages of the project. DeepForge will promote "data as product," "model as product," and "service as product" concepts through integration with the Digital Object Identifier (DOI) infrastructure. DeepForge will enable scientist to assign DOIs to their shared assets providing data provenance enabling citing and publicly reproducing research results by executing the referenced ANN workflows with the linked data artifacts.
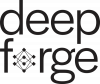